In our last blog post we took a look at a network map of companies in the HR Tech space. For that we used the “People Also Viewed” data for LinkedIn company profiles. After completing that analysis we had another idea - why don’t we use the same People Also Viewed but instead of for company profiles rather extract it from the profiles of individuals on LinkedIn? And that is exactly what we have done and summarized in this post.
As a company that focuses on the People Analytics space, one of the most interesting networks for us is the global People Analytics network, i.e. the network that helps understand the relationships and connections that exist between people who work in the People Analytics space globally. This post summarizes the approach we took to mapping this network using LinkedIn data and also walks through some of the key insights from this network. It’s not designed to be a comprehensive analysis of the network though and we are sure that others in this space will extract other insights. It’s for this reason that we’ve also provided links to the interactive networks in Polinode below.
Background and Our Approach
As in our previous post, we made the decision to use data from LinkedIn, specifically the set of “People Also Viewed” people available on a given individual’s LinkedIn profile page (please see the screenshot below).

We first needed to come up with a list of seed profiles to start the network data collection with. In contrast to our previous company-to-company network where we started with 150 seed company profiles, this time we decided to start with just four seed profiles of individuals. The four individuals that we chose were David Green, Heather Whiteman, Richard Rosenow and Stacia Sherman Garr. Of course we wanted to select well-known and influential people in the People Analytics community but we also wanted some diversity in the seed profiles that we selected - David Green is based in the UK, Heather Whiteman has strong connections to academia, Richard Rosenow is US-based and works at a vendor (One Model), and Stacia Sherman Garr works at a leading People Analytics Research / Analyst firms (Red Thread Research). So, all are connected to the People Analytics space, but in different ways.
So, the first step was to extract the People Also Viewed data for these four seed profiles and, at the same time, we also captured other attributes from their LinkedIn profiles such as their occupation, the country they live in, the number of followers they have, and so on. The next step was to iterate on this process, i.e. to repeat it for the profiles in the People Also Viewed data of the seed profiles. We continued this process until a specified number of profiles had been collected, 500 people was the stopping condition in this case. Once the data had been collected, it was processed into a network format (i.e. nodes and edges with attributes on each of those nodes and edges) and uploaded to Polinode via the Polinode API.
Each node of course represents a distinct individual and two people are connected in the network if one person was listed in the set of People Also Viewed data for the other person’s profile.
A Short Reflection on the Data and its Limitations
Most people who work in People Analytics are very familiar with the importance of good quality data and also that there is, almost invariably, some limitations of the data that you have available. This set of data and analysis is no different. It’s important to note that if we had used a different group of seed profiles we would of course get a different result. There will also be some people that play an important role in the People Analytics community who will not appear in this network or whose position is less central than they may expect or that in reality may be the case. Also, a small number of individuals have turned off the People Also Viewed feature in LinkedIn and so will not appear in the network for that reason or will only have incoming connections. Privacy controls like this are an important feature that LinkedIn makes available and it would be remiss of us to not point out their impact on the analysis. They do, however, impact only a small single-digit percentage of the profiles that we collected (2.4% to be precise).
Another point that we feel it is important to not lose sight of is that this data is quite different to say nominations data from an Active ONA. It's different because the individual does not control or report on their connection with others via the People Also Viewed data. It's still a very interesting dataset but we shouldn’t view these connections like nominations in an Active ONA or like communication data in a Passive ONA. The nature of these edges is quite different.
Lastly, some of the individuals in the network had multiple current positions, e.g. VP of People Analytics and Advisory Board Member. For these individuals, we used some heuristics to try and pick their primary current role. Still, there will be some imperfect data for individuals with multiple current positions and we extend our apologies to anyone in this category.
The Overall People Analytics Network
Now we understand how the data has been collected, let’s take a look at the resulting network. The raw, unfiltered network includes a total of 4,742 individuals connected by 8,108 relationships. It's worth noting that the retrieved data encompasses more than the 500 individuals we mentioned previously as complete profiles weren't obtained for every person due to the stopping condition. On average, each individual has 3.4 connections. To reduce noise in the network, we've filtered out those individuals with less than two connections in the visual representations below, resulting in a core network of 888 people connected by 4,258 relationships.
The image below shows this core network colored by the country the individual is located in. We have also sized the nodes in the network here by the total number of incoming connections of each individual, i.e. the number of times they were identified as a People Also Viewed individual. Additionally, we’ve simplified the list of countries by combining countries with less representation into an “Other” category.
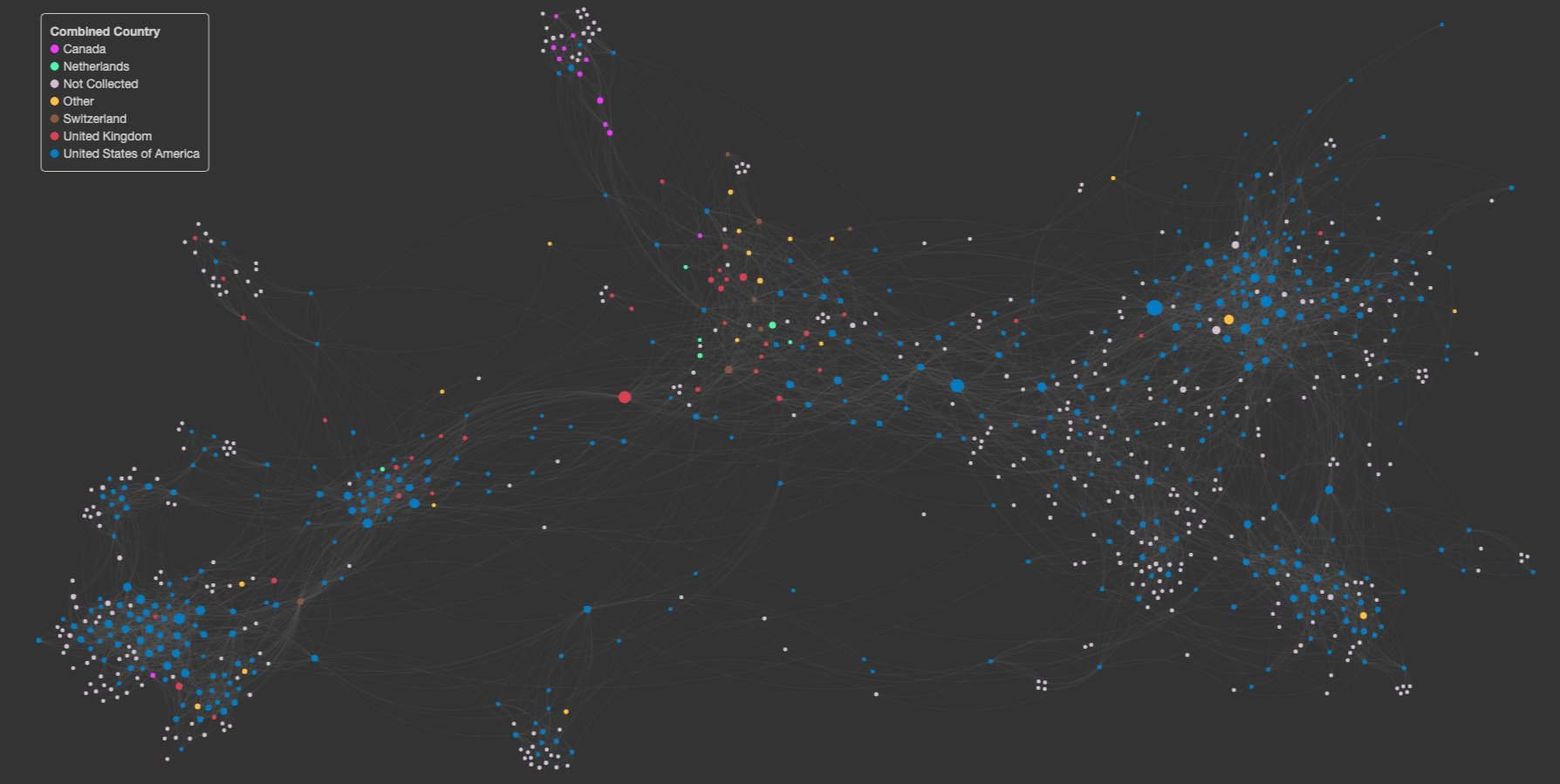
Though a large proportion of individuals in the network above are based in the USA, there are some parts of the network that are full of people from other countries. For example, the center of the network includes a geographically diverse mix of individuals from the United Kingdom, Switzerland, the Netherlands, France, Spain, Germany, Japan and Singapore, as well as the USA. Additionally, the group above this center network includes individuals mostly based in Canada that work for Visier, a leading People Analytics technology company that is headquartered in Canada.
We have saved multiple views in Polinode for this network and the image below shows another view that we have saved - one where we have colored by whether or not an individual was in the seed list. All of the seed profiles are relatively close to the center of the overall network. For example, Richard Rosenow, VP of People Analytics Strategy at One Model, sits between the center and right side of the network, which mostly includes individuals at large tech companies like Meta, Google, LinkedIn, Twitter and Salseforce. Positioned between the center and left side of the network is David Green, who is the Executive Director and Member of the Board at Insight222. If you've already accessed the interactive network by clicking the link above, you've probably observed significant clustering or grouping of individuals based on factors other than just country; we’ll be looking at that very soon.
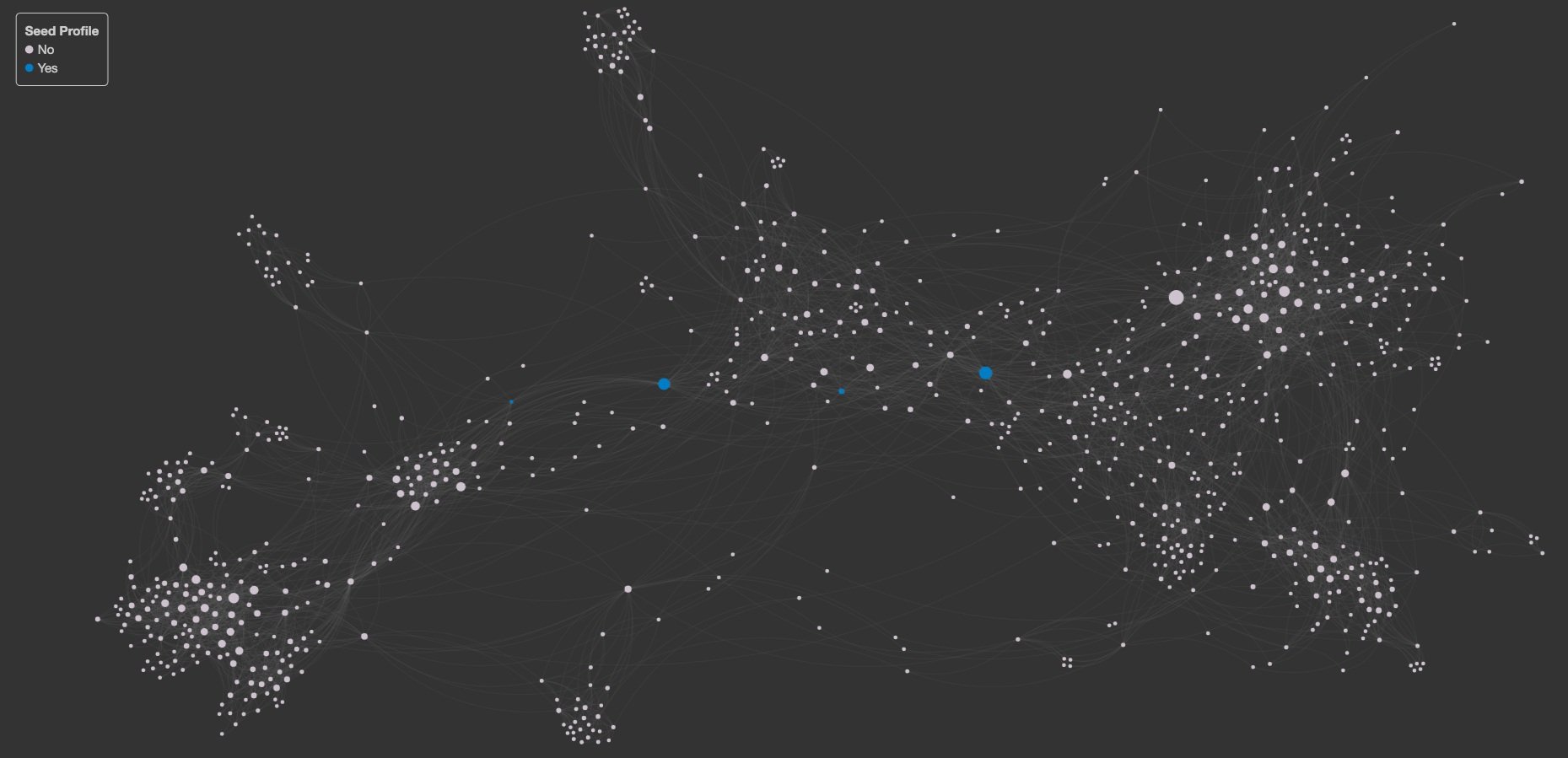
To better identify the clusters in the network and understand the mix of people in each, we ran a community detection algorithm in Polinode, which finds non-overlapping groups of relatively connected individuals. Several methods are available for detecting these groups, each offering tradeoffs in speed and quality. Arguably the most commonly applied algorithm is the Louvain method for community detection and that is what we used.
The following image shows the network colored by the resulting communities.

The result of the above was the detection of 11 communities among the 888 people in the filtered network. If you hover over a few individuals in the interactive network, you will notice that similar individuals tend to be grouped together. In the next section we dive a bit deeper into a few of these communities.
A Closer Look at Six Different Groups in the Network
We have chosen six groups to look at in more detail. They are what we have labeled in the image below the Meta community, the Google community, the LinkedIn community, the Microsoft community, the Visier community, and the HR & General Influencers community. Certainly, not every individual within these six communities fits perfectly into these categories. We are using a bit of a shorthand here. That said, it's fascinating to look at which individuals have been assigned to these communities while not really conforming to those categories - sometimes the exceptions are as interesting as the rules.

The first group we’ll look at is the Meta community at the top right of the network. It includes individuals like Ben Teusch, People Analytics Manager at Meta, and Josh Wondra, People Research Scientist at Meta. Though Meta employees make up the majority of this community, there are a mix of other companies represented as well like Uber, Airtable, Lacework and Indeed. The following image gives a closer look at this community. We have added labels next to some of the more connected individuals in the group.
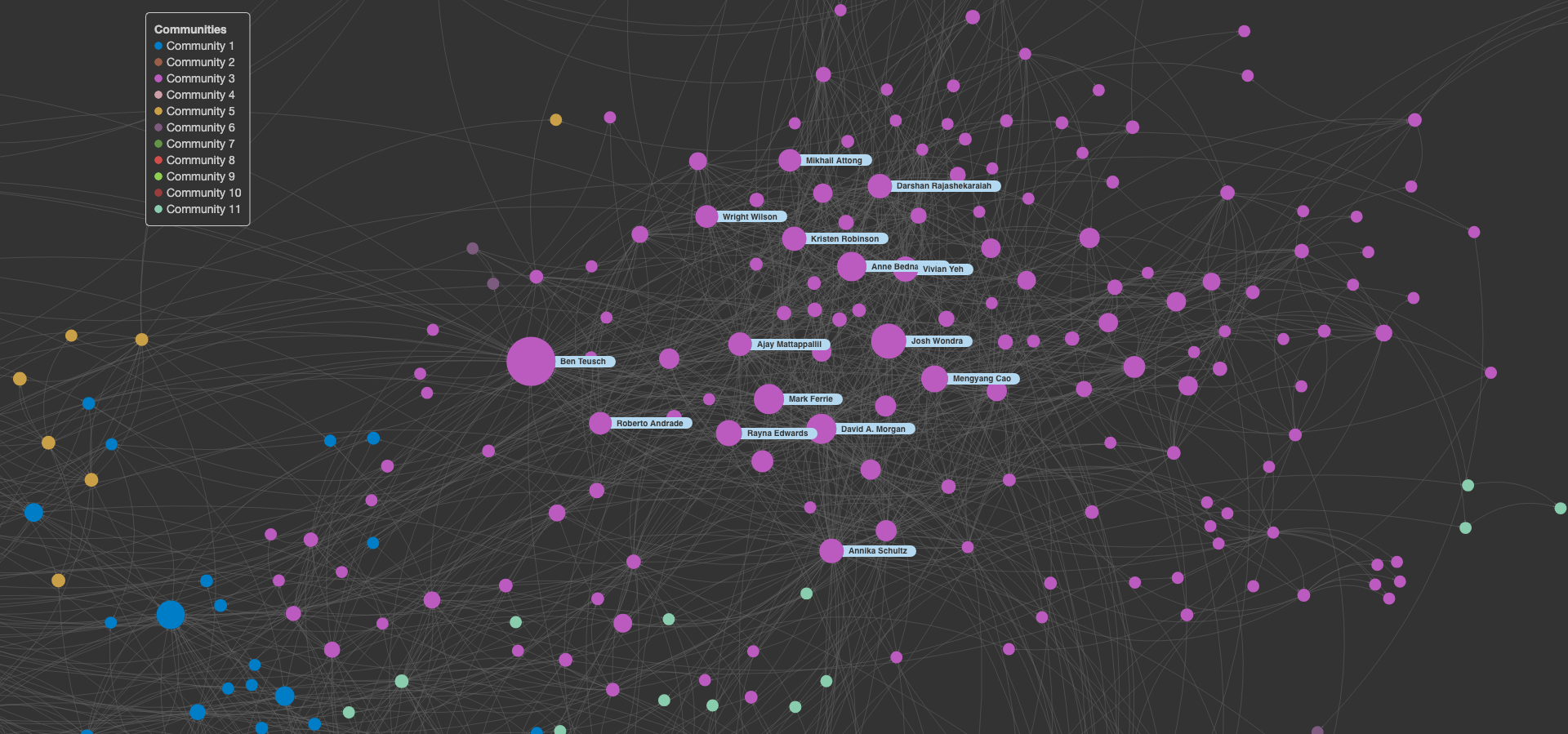
Just below and to the right of the Meta community is the Google community. Similar to the Meta community, it mostly includes Google employees in People Analytics roles. It also includes some previous Google employees that now work at Meta. For example, Christopher Patton, People Analytics Manager at Meta, sits between the Google and Meta communities. He was previously with Google, so it’s interesting to see his Google connections persist after moving to Meta. We often see that in Active and Passive ONA’s too. That is to say, when a person has left a team or division and moved to a new one they typically retain important links from the previous group. Some organizations that we have worked with use internal mobility very strategically to promote these kinds of bridging connections.

Positioned relatively close to the Google community is the LinkedIn community which we’ll take a look at next. Of course it’s quite meta to be using LinkedIn data to analyze the People Analytics community that then includes a strong group of People Analytics practitioners from LinkedIn within it! Though these employees from LinkedIn make up a significant component of this group, there is a more diverse mix of companies than the first two groups we looked at. We’ve highlighted the main group of LinkedIn employees in the labeled network image below. However, there are some people in the labeled group who work outside of LinkedIn like Christopher Praley, Manager, People Analytics at Thumbtack. Companies like Takeda are also represented by individuals like Allie Belier, People Analytics Consulting Lead at Takeda The image below shows a close-up view of the LinkedIn community and you will notice how the actual LinkedIn employees appear towards the bottom right with the employees from other companies positioned more towards the left, i.e. closer to that central blue Community 1.
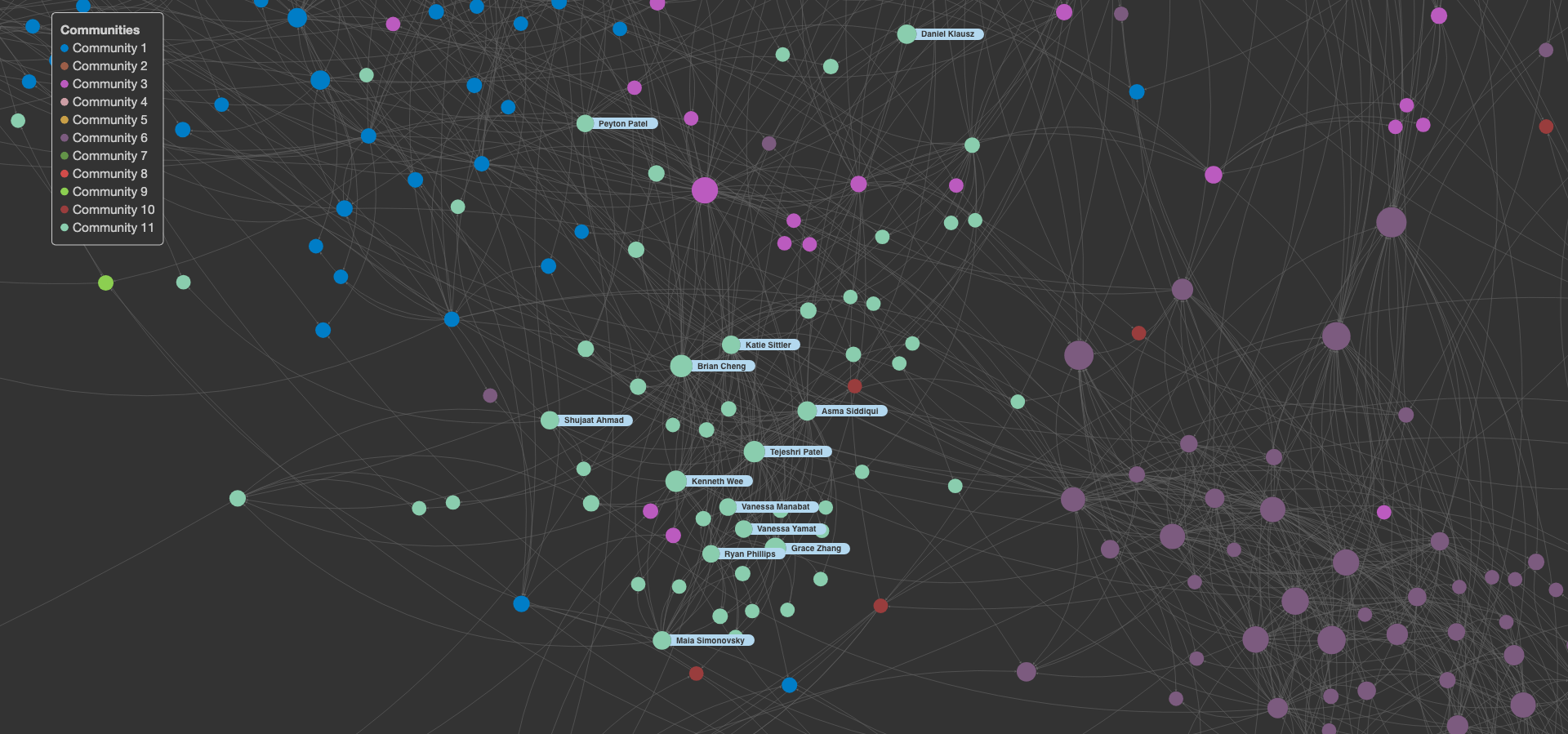
The next community we’ll cover is the Microsoft community. This group is a subgroup of Community 1. Included in the Microsoft community are individuals like Panos Panay, Chief Product Officer, Jason Zander, Executive Vice President, and Lindsay-Rae McIntyre, Chief Diversity Officer at Microsoft, respectively. Two of the key bridges between the Microsoft community and the rest of the network are Kathleen Hogan, Chief People Officer, and Dawn Klinghoffer, Head of People Analytics at Microsoft, respectively. The image below shows a close-up view of the Microsoft community. It makes sense that Dawn Klinghoffer sits in between the broader, more central People Analytics community in the center of the network, and this Microsoft community where most people work in People Analytics adjacent roles rather than within People Analytics teams.

The Visier community towards the top left of the overall network also sits somewhat apart from the core of the network. What is likely happening here is that both Microsoft and Visier provide products to People Analytics teams and so some of the company is connected to the broader community even though those individuals might not work directly in People Analytics itself. But within each of these companies, we do see some individuals that sit very much within the People Analytics company but are still connected back to their respective companies. In the case of Microsoft, that was Dawn Klinghoffer. For Visier, these individuals are Ian Cook, VP, Research and Strategy at Visier, and Lexy Martin, Principal, Research and Customer Value at Visier. Both Ian and Lexy sit close to the somewhat separate Visier community but are still very much in that core, central blue community. The image below shows a close-up view of the Visier community, noting of course that Ian, Lexy and a few other Visier employees sit outside of that Visier community while still being very much connected back into it.

Lastly, we’ll look at the HR & General Influencers community. As indicated by the name we have used, this group has two subgroups that are well connected. The HR Influencers subgroup, shown in the image below, includes individuals such as Josh Bersin, Founder and CEO at The Josh Bersin Company, who has an interest in People Analytics but is not exclusively focused on it. His research and analysis tends to be more general in nature, directed at the overall HR Tech space. There are also Recruiting influencers such as William Tincup. So, these are individuals in the broader HR Tech space.
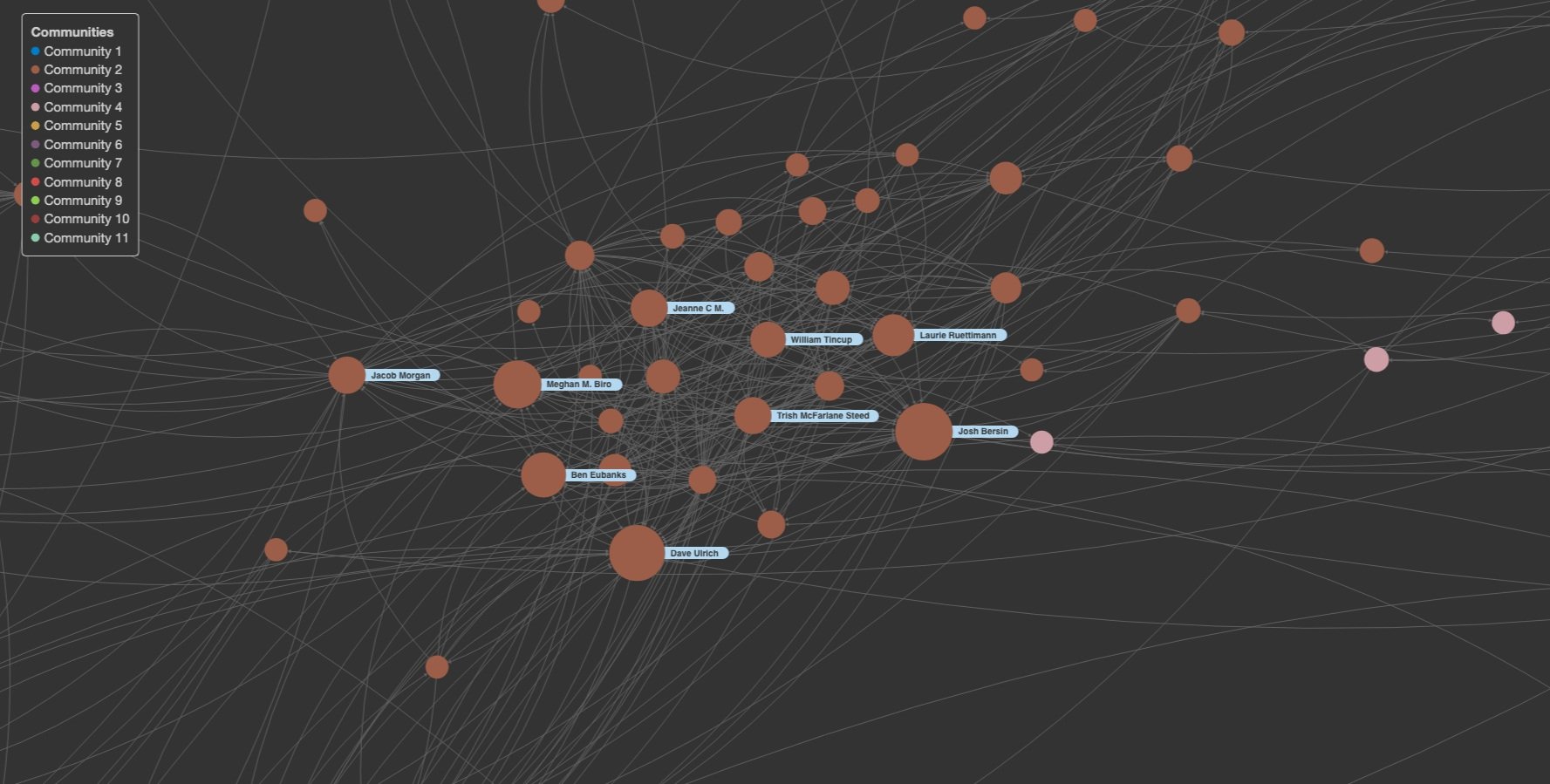
The second subgroup in the HR & General Influencers community are the General Influencers. This subgroup includes several well-known personalities beyond the HR and People Analytics domains like Tim Ferriss, Brené Brown, Simon Sinek, Tony Robbins, Jamie Dimon, Mike Bloomberg, Kevin O' Leary and Oprah Winfrey, as well some well-known actors like Robert Downy Jr. and Ryan Reynolds. Also in this group are key Microsoft figures, Bill Gates and Satya Nadella. Unsurprisingly, Satya Nadella sits between this community and the Microsoft community.
This group is of course quite different to the rest of the network. It looks like this group emerged as (a) individuals in it are generally very well known and likely have a great many of connections in the overall LinkedIn network (not just the subset we are focussed on) and (b) a handful of People Analytics influencers also have a broader public appeal which likely led to the inclusion of this group. Consider Adam Grant as an example. He is an author of a number of books that align with the domain of People Analytics and performs a significant role at the Wharton People Analytics conference. As such, he receives incoming edges from leaders in People Analytics such as Heather Whiteman. But his work also likely has broader appeal and his outgoing edges connect to individuals like Simon Sinek and Marshall Goldsmith.
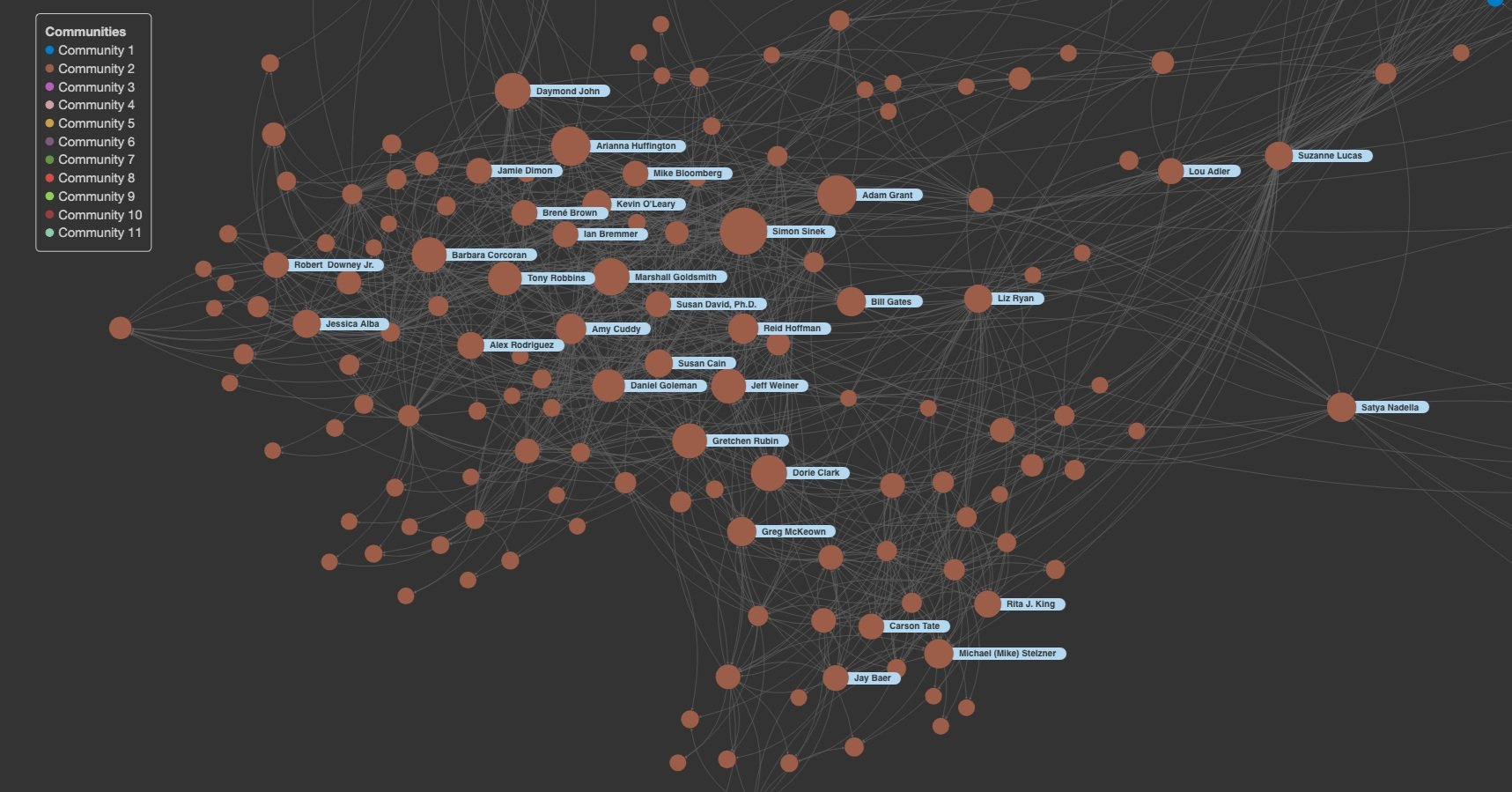
We’ve looked into a number of the communities in the network above and have also mentioned quite a few individuals. Next we’ll look at a couple of the individuals mentioned above in a bit more detail, Ben Teusch and Richard Rosenow.
Looking at Ben Teusch and Richard Rosenow in More Detail
We chose these two individuals because they are two of the most connected individuals in the overall network. Let’s look at Ben Teusch, People Analytics Manager at Meta, first. He has a total of 47 incoming connections. He previously held People Analytics roles at Capital Group and hiQ Labs. Given his current role with Meta, it makes sense that he is a member of the Meta community. In the network, he is positioned between the Meta community and the center of the network, Community 1, in a bridging position relative to those two groups. Some of his connections outside of Meta include two of the four seed profiles, Richard Rosenow and Heather Whiteman, as well as Susan Biancani, Director, Talent Analytics at Netflix and Emory Clayton Johson, Director, People Analytics at Salesforce. His connections are relatively diverse, spanning four of the communities in the overall network. The image below shows the ego network for Ben Teusch, i.e. the connections to and from him as well as the connections between the individuals that are connected to him.
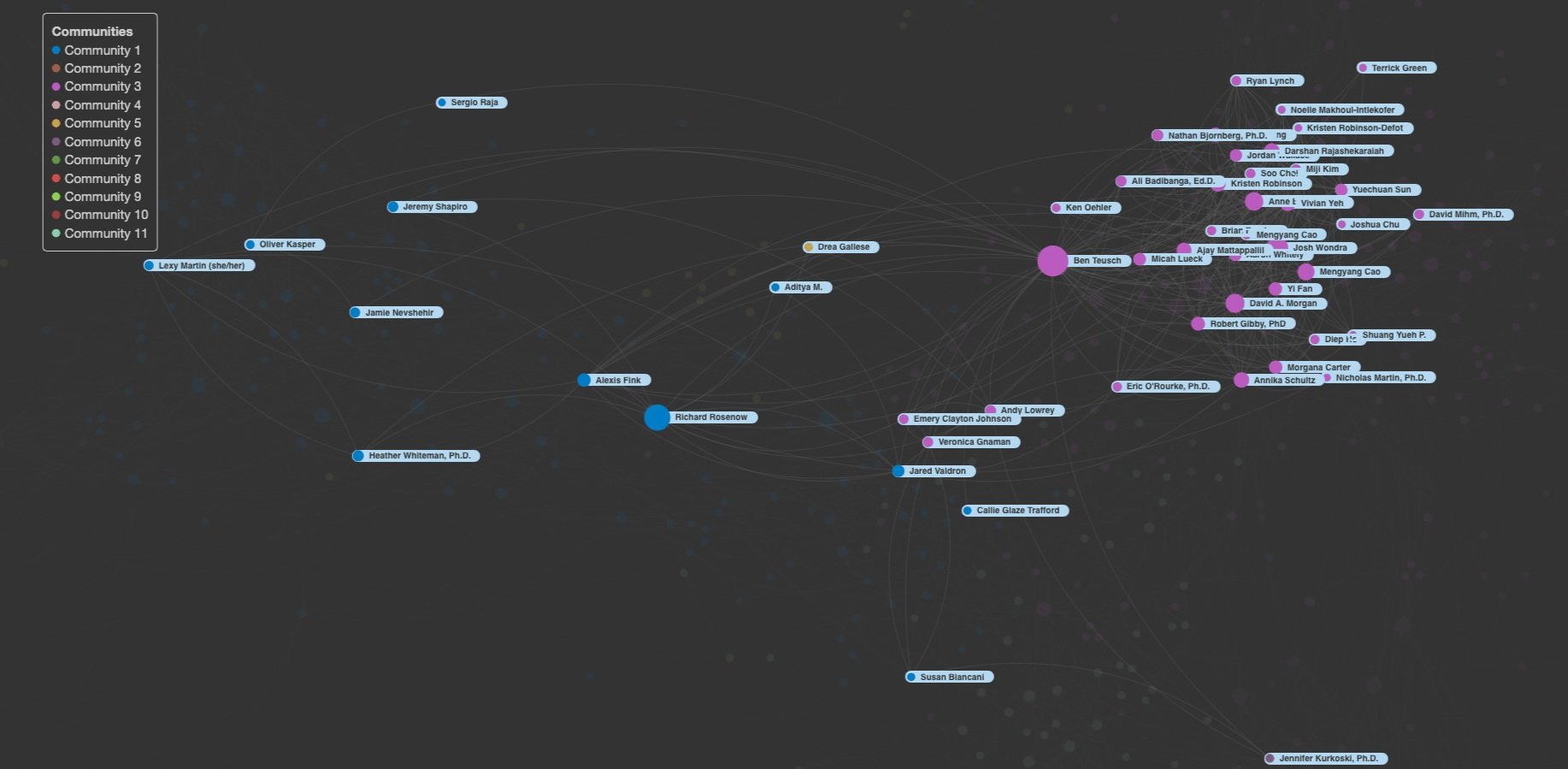
The second individual we’ll cover is also one of the seed profiles, Richard Rosenow, VP People Analytics Strategy at One Model. He has a total of 38 incoming connections. Slightly less than Ben Teusch but, as we’ll see, his network is arguably even more diverse. In addition to his work at One Model, Richard has previously worked at Argo AI, Nike, Uber and Facebook. As mentioned above, Richard sits between Community 1, which he is a member of, and the right side of the network with some of the larger tech companies like Meta / Facebook, Google, LinkedIn, Twitter and Salesforce. Similar to Ben Teusch, his connections encompass four of the communities in the overall network. Some of Richard Rosenow’s connections include two of the seed profiles, David Green and Heather Whiteman, as well as Dawn Klinghoffer, Head of People Analytics at Microsoft, and Caitlin Hogan, People Analytics Team Lead at Google. The image below shows the ego network for Richard Rosenow, i.e. the connections to and from him as well as the connections between the individuals that are connected to him.

Conclusion
The community and individual examples presented above offer just a glimpse into the wealth of insights that this kind of LinkedIn network data can provide. If you're enthusiastic about delving deeper into this network and uncovering more, we encourage you to explore the interactive visualizations available on the Polinode platform via the links provided above. Additionally, please don't hesitate to contact us at info@polinode.com if you would like to chat about this network or anything else live.