When it comes to Organizational Network Analysis (ONA) it’s common for organizations to reach for the traditional sources of data: they either run an Active ONA where they ask employees questions about their working relationships with others or they tap into the more common passive data sources such as metadata from emails, meeting invites and enterprise social networks such as Slack or Teams. At Polinode we are used to using this kind of data to help organizations understand how they are really functioning and also to improve in areas such as collaboration, DE&I, identifying talent and change management. But this is by no means all of the data that we work with. In fact, pretty much every organization (both large and small) has, in our experience, untapped sources of relationship data over and above these more common data sources. The purpose of this post is to highlight some of these less commonly utilized data sources.
Why is this important? Because often it can be easier to tap into these alternative data sources than, for example, email data. And these data sources can offer really valuable insights for what is often a relatively small effort.
In the following sections, we’ll cover seven of these overlooked data sources, each offering unique perspectives and opportunities for analysis. From 360 reviews to skills data, each source provides valuable insights that can deepen our understanding of organizational networks and the way that an organization is functioning (or not!).
360 Reviews
Many organizations make use of an online platform to run their annual (or sometimes more frequently) performance review process. Typically individuals are asked for a series of nominations, i.e. to select a set of reviewers. Those reviewers are then approved and sometimes additions or deletions are made before they then provide both quantitative and qualitative feedback. Review processes come in many different forms and this is by no means universal. The key though is that where an individual has a significant amount of input into who is reviewing them then that nomination data can be a fairly good proxy for the actual working relationships in an organization. Without a doubt, the nomination process itself can introduce a fair bit of bias (we can likely all think of at least one individual that others may avoid seeking feedback from) but, we will return to that issue shortly. For the moment, we just want to focus on utilizing this frequently overlooked source of network data.
So, whether you are using one of the larger providers such as Lattice, 15Five, Betterworks or Culture Amp that offer not only comprehensive platforms for collecting feedback but are also now increasingly offering robust employee engagement solutions (Lattive even offers an HRIS now!) or you are using one of the arguably smaller and more nimble upstarts like Small Improvements you likely already have this data and, we suspect, not using it to really understand things like collaboration patterns across the company. It’s also worth mentioning providers of 360 assessments here such as Hogan and SHL. Generally it’s harder to analyze data from those kinds of sources but, with the appropriate safeguards, it’s certainly possible.
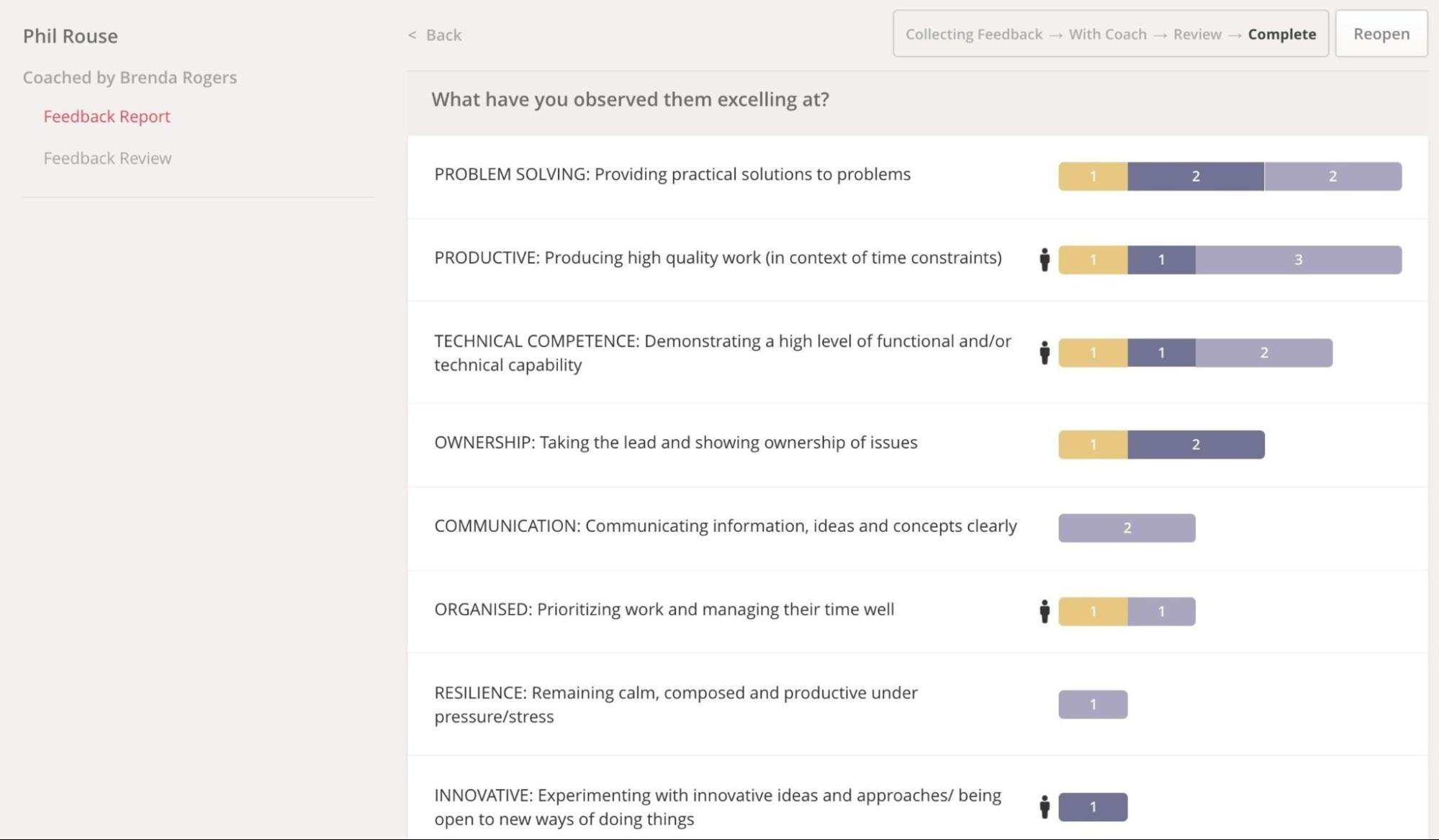
One specific application of 360-degree review data in organizational network analysis (ONA) is the identification of influential individuals within the network. By analyzing feedback from peers, managers, and direct reports, organizations can pinpoint these influencers. And by “influencers'' we don’t just mean employees that are nominated more than we would expect - we also mean individuals that bridge different groups in the organization and even, to borrow from Douglas Guilbeault and Damon Centola’s recent work, individuals that have a high degree of complex centrality.
Finally, we promised to come back to that question of bias in nominations in the 360 performance review process. Well, it probably won’t come as a surprise to you that one way that we can address this (as well as the issue of the burden of the review process falling disproportionately on a small number of individuals) is to actually use network data. That is to say, that we can use other sources of passive data such as email metadata and meeting invites to help inform the reviewer nomination process.
Peer-to-Peer Recognition Tools
A few years ago there was a lot of discussion in the HR Tech space about the “end of performance reviews”. Performance review cycles of course didn’t end, at least not at most organizations. Discussions are becoming far more focussed on development and less quantitative in nature. There has also been an emphasis on more frequent data collection and more frequent feedback. One of the categories of tools that has grown in popularity and, we would argue, partly as a result of this shift in mindset has been peer-to-peer recognition tools. Again, we would like to give you a couple of examples to illustrate the kind of tool and data that we are talking about - two of the most notable companies in this space are Workhuman and Bonusly.
Peer-to-peer recognition platforms can play a crucial role in fostering a culture of appreciation and collaboration within organizations. These platforms provide employees with the opportunity to recognize and appreciate their colleagues' contributions, creating networks of appreciation that extend across teams and departments. But, for our purposes, they are also sources of incredibly powerful and often near real-time network data.
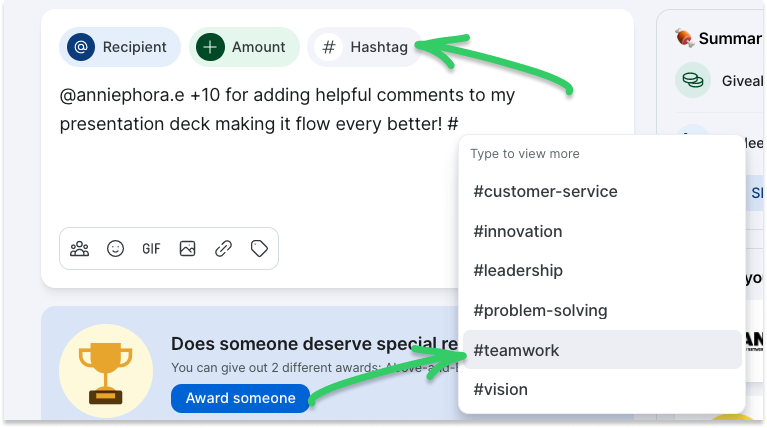
Peer-to-peer recognition platforms offer a unique lens through which organizations can explore collaboration dynamics and uncover informal networks within the organization. By delving into recognition patterns and interactions on these platforms, organizations can gain valuable insights into how employees collaborate, communicate, and interact across teams and departments. These insights extend beyond formal hierarchies, revealing hidden connections and even emerging leaders within the organization. Ultimately though, like all sources of network data, the data quality is important - there needs to be real value associated with the recognition and if there is a culture of using up or distributing unused recognition points without sufficient thought then the data is likely to be less valuable than would otherwise be the case. In other words, these platforms are rarely just set and forget, some active maintenance and support is required.
Opportunity Marketplaces
Opportunity marketplaces (also called Talent Marketplaces) have emerged as important platforms within organizations, facilitating internal talent mobility and career development. These platforms enable employees to explore new roles, projects, and learning opportunities tailored to their skills, experience, and career aspirations. By creating transparent and (hopefully) merit-based systems for talent mobility, opportunity marketplaces foster a culture of continuous growth and development, driving innovation and agility while enhancing employee engagement and retention.
Gloat, Beamery, and Fuel50 are among the top providers of opportunity marketplace platforms. Increasingly these platforms are leveraging AI-driven algorithms to match employees with relevant opportunities based on their skills and career goals, empowering organizations to optimize talent mobility and drive innovation.
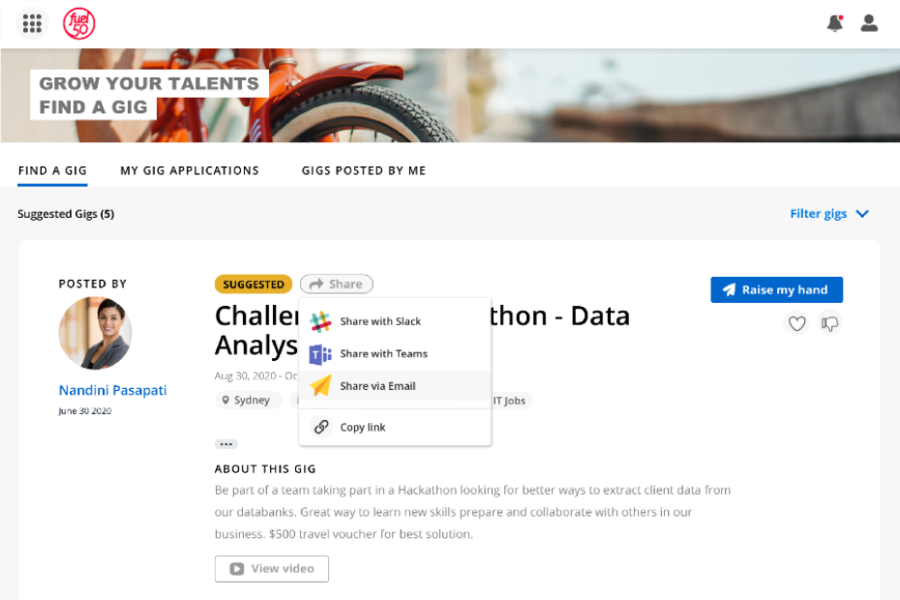
These opportunity marketplace platforms collect a number of sources of network data - for our purposes, we are particularly interested in the ability for employees to post and apply to short-term Gigs. This creates a global collaboration network, one that is often mostly self-organizing and where Organizational Network Analysis can be incredibly valuable, making these self-organizing networks visible and able to be analyzed, understood and even nurtured. Of course these larger players in the Opportunity Marketplace space are not solely focussed on it. There is a natural linkage between these kinds of Opportunity Marketplaces and skills.
In addition to these more specialized platforms, several HRIS systems also offer opportunity marketplaces, including SAP SuccessFactors, Cornerstone, and Workday. Recently, we collaborated with Hemerson Paes, Senior Global Network Catalyst at Roche, leveraging opportunity marketplace data from the Workday Opportunity Marketplace for an organizational network analysis. To foster innovation and adaptability within the organization, Roche empowers employees to, where appropriate, work on short to medium term projects known as gigs. In essence each gig has a host and employees can apply to join a gig if they feel like their skills (available on their profile) are a match for the requirements of the gig (also listed on the platform). Roche leveraged the data on employees and their involvement in gigs to construct a global network showing these collaborations, many of which bridge different countries and teams / functions. For a deeper dive into the project and to learn about the insights gleaned from the analysis, you can listen to Hemerson's detailed discussion on the Directionally Correct podcast.
Work Patterns Analysis
Understanding how employees collaborate, engage, and work together is crucial for optimizing organizational performance. Tools like Microsoft Viva Insights and Worklytics are a couple of the more recognizable players in this space, providing organizations with insights into work patterns that can drive strategic decisions.
Work patterns analysis involves examining various aspects of employee behavior, such as communication patterns and collaboration dynamics. By analyzing data from sources that include email, calendar, chat, and project management tools, organizations can uncover valuable insights into how work gets done. For example, analyzing email and meeting volumes outside of standard work hours can provide insights into employee engagement and potential burnout. Understanding these work patterns enables organizations to implement strategies to mitigate burnout, improve work-life balance, and enhance overall employee well-being.
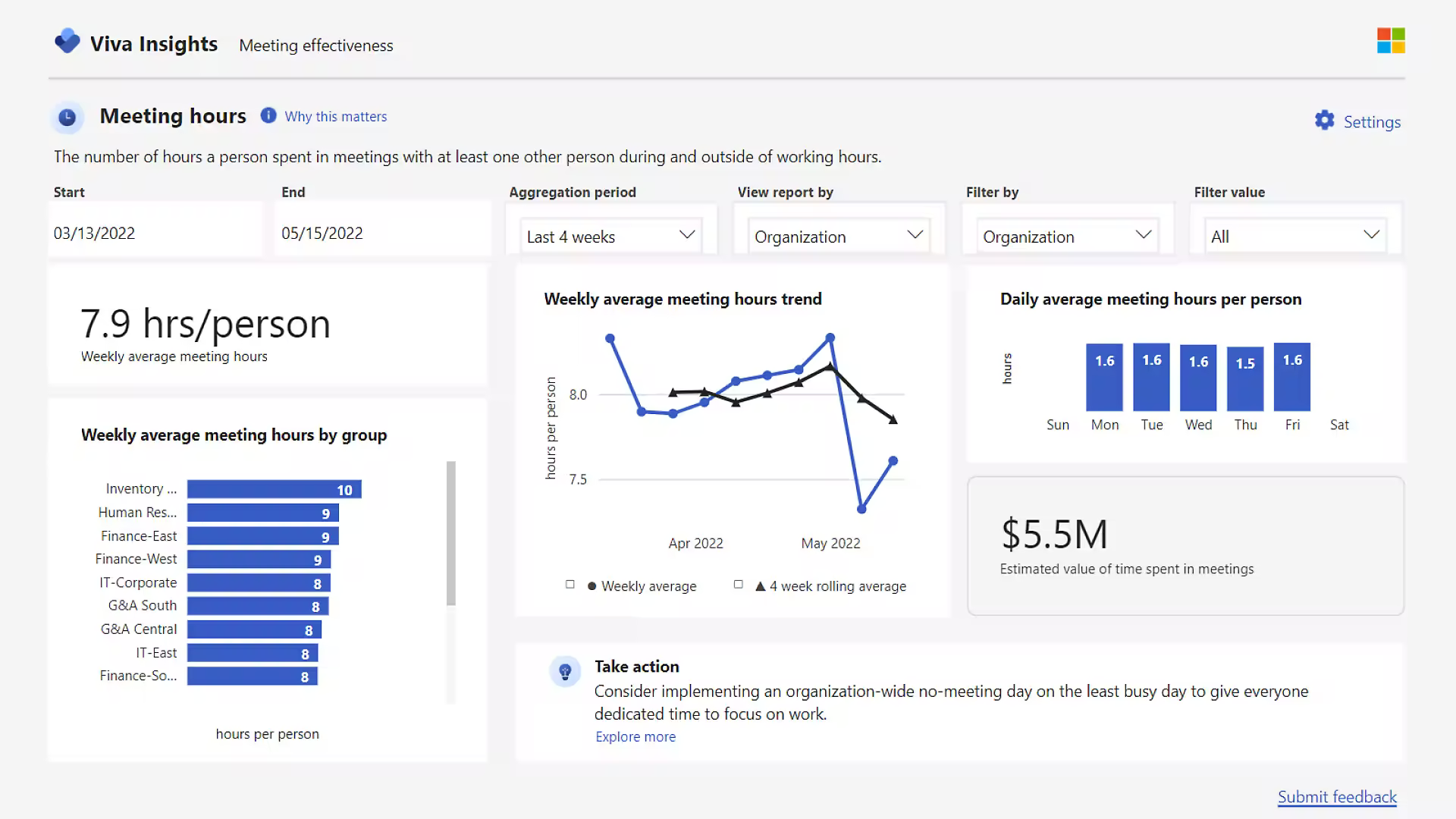
There are a couple of important connections between Work Patterns Analysis and Organizational Network Analysis. The first is that the passive data that is collected for Work Patterns Analysis is also often used to understand the collaboration between groups in the organization (or even, in some circumstances, between individuals). There are a number of Polinode customers who export collaboration matrices from Viva Insights and then import them into Polinode to use our interactive network analysis and visualization to further analyze the data from a network perspective (beyond the inbuilt tools that Viva provides). We also have some customers who use our built-in passive data integrations with Office 365 and Google Workspace for work patterns analysis. These integrations are designed to make passive Organizational Network Analysis but we also give customers the ability to download the raw message-by-message data (anonymised where appropriate) for the purposes of work patterns analysis.
Additionally, work patterns analysis can complement organizational network analysis by providing context for network dynamics. For instance, combining data on communication patterns with ONA insights can help identify organizational bottlenecks and inefficiencies. By understanding how work patterns influence network structures and vice versa, organizations can optimize team collaboration, streamline workflow processes, and foster a more connected and productive workforce.
The bottom line is that it’s a two-way street - data that has been collected for Work Patterns Analysis can be used for ONA and data that is collected for passive ONA can often be used for Work Patterns Analysis.
Talent Intelligence Data
In today's interconnected world, talent intelligence platforms play a crucial role in providing insights into industry-wide movements and connections. Companies like Lightcast, Gartner TalentNeuron, and Public Insight offer valuable data and analytics to inform competitive talent strategies and workforce planning decisions. These platforms provide insights into talent dynamics, industry trends, and workforce movements, enabling organizations to make data-driven talent decisions.
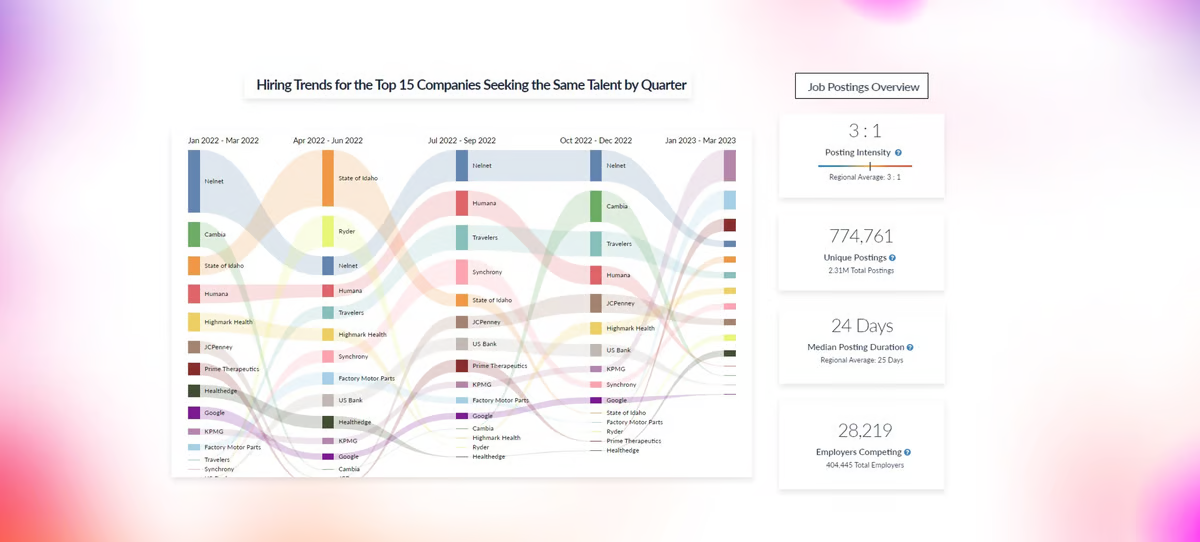
The awareness of the application of network analysis to Talent Intelligence Data is certainly lower than it is for some of the other sources that we previously mentioned such as Work Patterns Analysis data. So let us give you a couple of examples to demonstrate. One compelling use case for utilizing talent intelligence data is the analysis of company-to-company movements. By examining talent movements between companies within an industry, organizations can gain insights into the broader network of talent connections and relationships. Understanding these movements can inform recruitment strategies, identify emerging trends, and uncover potential partnership opportunities. Additionally, analyzing geographic talent migrations can provide insights into talent supply and demand dynamics, helping organizations anticipate talent shortages or surpluses and adjust their workforce strategies accordingly. Overall, talent intelligence data offers a valuable lens through which organizations can understand and navigate the complex network of talent relationships within their industry.
It's important to note, however, that data availability and the level of flexibility varies from provider to provider, so the data may be easier to obtain from some more so than others. For example, LinkedIn Talent Insights, another player in this space, offers a wealth of talent-related insights, but accessing the underlying data for deeper analysis can be challenging. Despite these hurdles, LinkedIn remains a valuable resource for understanding professional networks and industry landscapes.
LinkedIn Data
While we are on the topic of LinkedIn, it would be remiss of us to not mention some of the work that we have previously done utilizing network data. In our previous blog post titled "Mapping the Global People Analytics Network Using LinkedIn Data," we explored the global network of individuals within the People Analytics space using data extracted from LinkedIn profiles.
Our methodology involved leveraging the "People Also Viewed" feature on LinkedIn profiles to construct the network, starting with four diverse seed profiles: David Green, Heather Whiteman, Richard Rosenow, and Stacia Sherman Garr. These individuals were chosen for their influence and varied backgrounds, representing different geographic regions and roles within the field. We then expanded the network iteratively by collecting data from the profiles of individuals connected to our seed profiles, capturing attributes such as occupation, location, and followers. This process continued until we amassed data on 500 individuals, which was then transformed into a network format and uploaded to Polinode for further analysis. Through this approach, we aimed to uncover insights into the global People Analytics network, understanding its composition, dynamics, and influence within it.
The following image shows the network colored by the four seed profiles mentioned above.

Skills Data
We have already mentioned Skills Data in the context of Opportunity Marketplaces - there is a natural connection between the two. Skills has of course been a hot area in the HR Tech space for quite a few years now. Skills data provides valuable insights into the capabilities and proficiencies of an organization's workforce, serving as a cornerstone for effective talent management and strategic workforce planning.
In collaboration with one of our partners, we recently embarked on a project aimed at mapping the skill sets of employees within an organization. The project involved constructing a network of individuals and the skills they possessed. The insights derived from this network offered valuable perspectives on the skill landscape within the organization.
Utilizing the network of individuals and their skills, we further developed a co-occurrence network of these skills. In this setup, two skills were linked if a given individual possessed both of them, with the strength of the connection determined by the frequency of such co-occurrences across all individuals. Analysis of these networks unveiled clusters of co-occurring skills, often aligned with distinct job families or functional areas.
The insights garnered from this project shed light on the skill distribution within the organization and underscored the correlation between skill sets and job roles. This understanding has profound implications for talent management strategies, enabling companies to tailor recruitment efforts, training programs, and career development initiatives to align with the skill requirements of various job families.
Unfortunately we can’t share this network publicly but the following image illustrates an example of a skills co-occurrence network to demonstrate the idea.

Conclusion
Every day organizations are collecting an enormous amount of data that is natural network data and can be used for Organizational Network Analysis, i.e. to improve the way that organization functions through the power of network analysis. The purpose of this article was to touch on a few of the less well understood sources of network data that can be incredibly powerful. One of the nice things about the Polinode platform is that it is designed to be incredibly flexible and is capable of importing literally any type of network or relationship data. We have a lot of experience working with data sources such as the above so if you would like to chat about one of these data sources (or one that we haven’t mentioned) please reach out to us at info@polinode.com. We'd love to continue the conversation and help you unlock the potential of your organizational network data.